Structural analytics
The misunderstood challenge for modernising Risk and Finance technology
January 2025
Executive Summary
The banking sector is undergoing a profound transformation as organisations strive to modernise their data and analytics capabilities. Significant advancements have been made in digitisation, cloud adoption, and the initial implementation of artificial intelligence. However, modernising structural analytics – a cornerstone of Risk and Finance functions - remains a formidable challenge.
Structural analytics, which involves complex, predefined, and repeatable analytics, requires more than the flexible, exploratory tools that dominate the modern data and AI landscape. The lack of proven solutions tailored to these highly regulated workflows has left banks with two suboptimal choices: adapting general-purpose data science solutions or migrating legacy applications to the cloud. Unfortunately, neither approach has consistently delivered the business benefits achieved in other areas.
This white paper explores the challenges of modernising analytics architecture within Risk and Finance and argues that the prevailing notions of a “data-first” or “AI-first” organisation are too often misaligned with the needs of banking’s most analytically sophisticated functions.
Despite these challenges, there are substantial opportunities to improve the analytical, operational, and financial performance of the Risk and Finance functions through technology modernisation. However, realising these opportunities require innovative, purpose-built solutions—not a wholesale adoption of strategies that have proven successful in other contexts. This paper outlines a forward-looking approach to unlocking the full potential of modern cloud-based analytics infrastructure through deliberate, tailored solutions.
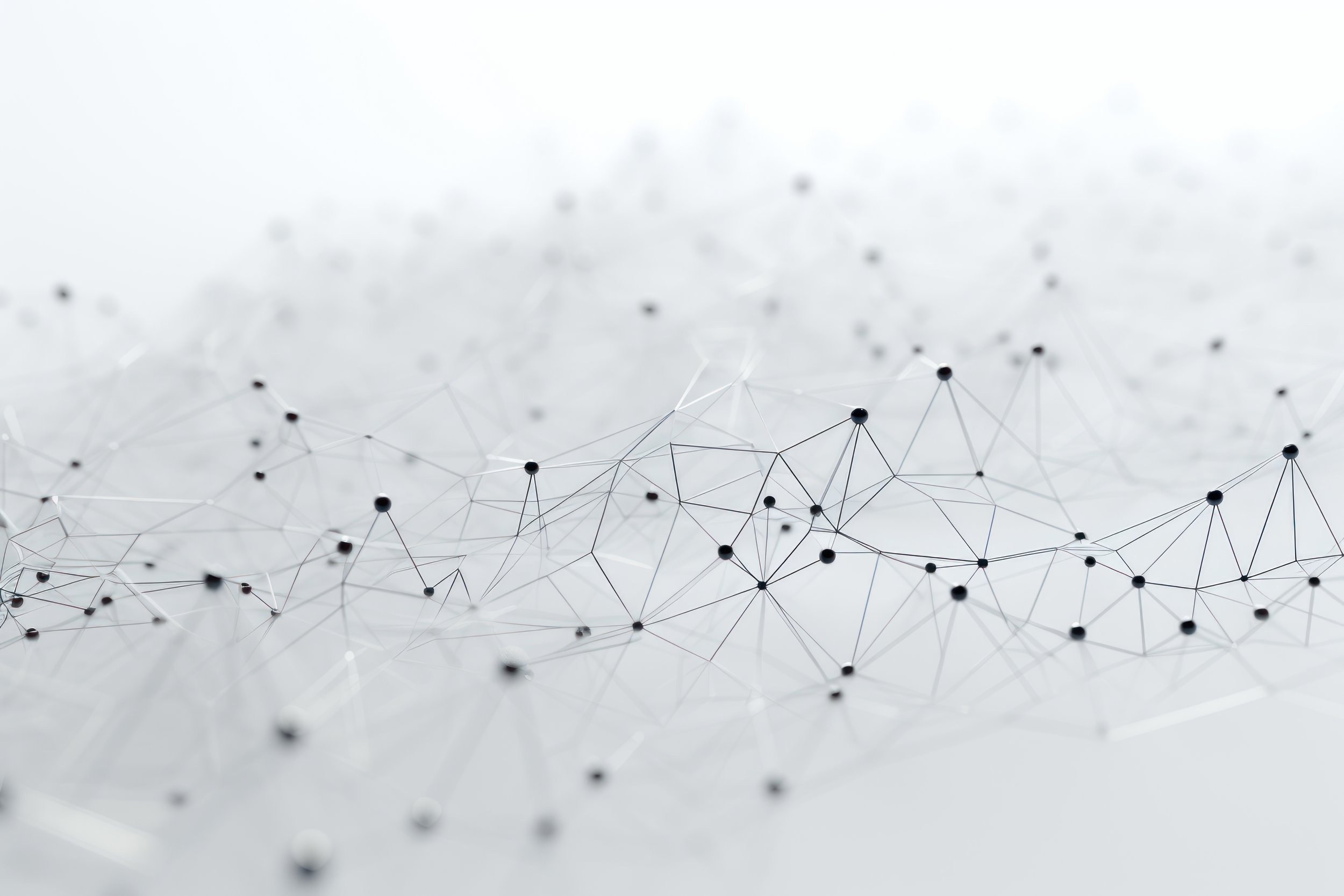
1. Banking’s three waves of data modernisation
Banking is said to be undergoing a fundamental transformation in how it manages data and analytics. This journey began with the digital revolution, which unleashed an explosion of new customer and operational data. It has since progressed with the widespread, though still incomplete, adoption of cloud technology. The next frontier—generative AI—promises even greater disruption, introducing radically new ways to understand and satisfy customer needs.
While the whole industry grapples with these changes, Risk and Finance - two of the most analytically intensive functions within the organisation—face unique challenges. Despite the promise of richer, faster, and more cost-effective analytics in the cloud, these benefits have been slow to materialise for these critical functions. Why is this the case?
It is because, unlike other departments, Risk and Finance are not well served by the architectural paradigms that have succeeded in other industries. Banks, in their drive to modernise, have often adopted packages of analytical tools which have established themselve as industry-agnostic standard. These tools are primarily designed for exploratory and generative analytics but overlook a third, fundamentally different mode of analytics: structural analytics, which is essential for regulated industries like banking.
This lack of explicit and innovative solutions for structural analytics explains why many Chief Risk Officers (CROs) and Chief Financial Officers (CFOs) remain underwhelmed by progress to date. Without more tailored solutions, these critical functions will continue to be underserved by the enterprise approach to analytics modernisation.
2. The critical importance of structural analytics in the banking sector
While analytics use cases are often categorised by the business problems they address or the methodologies they employ, it is perhaps more useful to consider three fundamental modes of analytics.
Exploratory analytics focuses on identifying patterns, trends, and relationships within data to inform future decisions or hypotheses. This open-ended, iterative, and human-led process involves techniques such as data mining, clustering, and visualisation. Analysts need the flexibility to experiment, pivot, and test assumptions, which is why modern tools emphasise raw data interaction, rapid prototyping, and visualisation capabilities.
Generative analytics leverages data to create new models, simulations, and content using predictive models, machine learning (ML) and artificial intelligence (AI) algorithms, where the system adapts as new data is introduced. The latest generative analytic solutions enable users to train algorithms on very large, complex, and often unstructured datasets, prioritising scalability, pipeline automation and ease of model deployment.
Structural analytics applies predefined, semi-rigid analytical processes to data. These processes often involving multiple component models with distinct meanings, performance standards, and compliance requirements. While optimising individual model performance is essential, ensuring that the overall workflow is reliable, repeatable, transparent, and compliant is equally critical. Examples of structural analytics in Risk and Finance include regulatory capital, liquidity reporting, automated credit decisioning, and portfolio stress testing. Failures in structural analytics can result in regulatory penalties, reputational damage, and sub-optimal decision-making.
These three analytic patterns are not formally exclusive. Some use-cases may incorporate characteristics of all three and an ideal bank analytics architecture would incorporate tools which enabled each mode of working. It is clear however, that structural analytics use-cases are hard to manage using only tools which are designed for exploratory and generative purposes.
3. Modernising structural analytics, two flawed strategies
Although structural analytics is a coherent conceptual category, it has not traditionally been recognised as a unified set of use-cases that could benefit from common platforms. This is primarily due to two factors. First, the structural analytics spans multiple functions, creating organisational barriers to unified solutions . Second, existing platforms were often developed reactively in response to evolving regulatory demands. As a result, the infrastructure supporting these use-cases is often fragmented, inflexible, and costly to maintain. Modernisation could deliver significant benefits, including improved analytic performance, streamlined operations, and lower technology costs.
However, organisations commonly seen as being exemplars of being “data-led,” “analytics-led,” or “AI-led” rarely come from regulated sectors like banking, where structural analytics plays a critical role. This lack of proven, category-level solutions has forced banks to adopt one of two strategies, neither of which has been highly successful.
The Mirroring Strategy involves adopting enterprise-level solutions. While these tools excel at exploratory and generative analytics, they often lack the rigor, repeatability, and compliance features required for processes like regulatory reporting and stress testing.
The Migration Strategy focuses on transferring legacy platforms from on-premises data centres to the cloud, without fundamentally rethinking the underlying operating model.
Both strategies face significant challenges. Adapting enterprise platforms designed for training AI models to support regulatory capital calculations or enterprise stress testing requires extensive customisation, which is resource-intensive, uncertain, and costly. Conversely, migrating legacy systems to the cloud risks perpetuating existing inefficiencies while incurring substantial costs and diverting resources from other critical transformation initiatives.
4. A potential way forwards
As the banking sector continues to modernise its data and analytics infrastructure, structural analytics will play a critical yet often under-appreciated role. While many banks have made strides in adopting cloud technologies and advanced data science tools, these solutions alone are insufficient. To fully realize the benefits of modernisation, Risk and Finance need innovative, purpose-built solutions to bridge the gap between established tools and the unique demands of their workflows.
These solutions must excel in five key areas:
Providing a standard framework for process orchestration which can be applied across models, functions, and processes
Systematically manage versioning, lineage, and auditability at both the model and workflow levels.
Enabling the rapid yet secure deployment of new models, data, and process changes, in a highly controlled and auditable manner.
Automating key governance documentation, including for model deployment, data lineage, and internal audit.
Enabling experimental model runs, production runs, post-run analytics, and model monitoring to be supported in one place, with shared model code, data, and infrastructure.
Solutions with these capabilities would allow the proliferation of model-specific platforms to be replaced with a single set of horizontal capabilities, whilst also reducing the burden on user groups to manually evidence compliance. Notably, this is a very different list of capabilities than the ones which data science platforms traditionally prioritise. Given the sector-specific nature of these requirements, development will likely require industry-driven innovation or close collaboration between banks and their technology vendors.