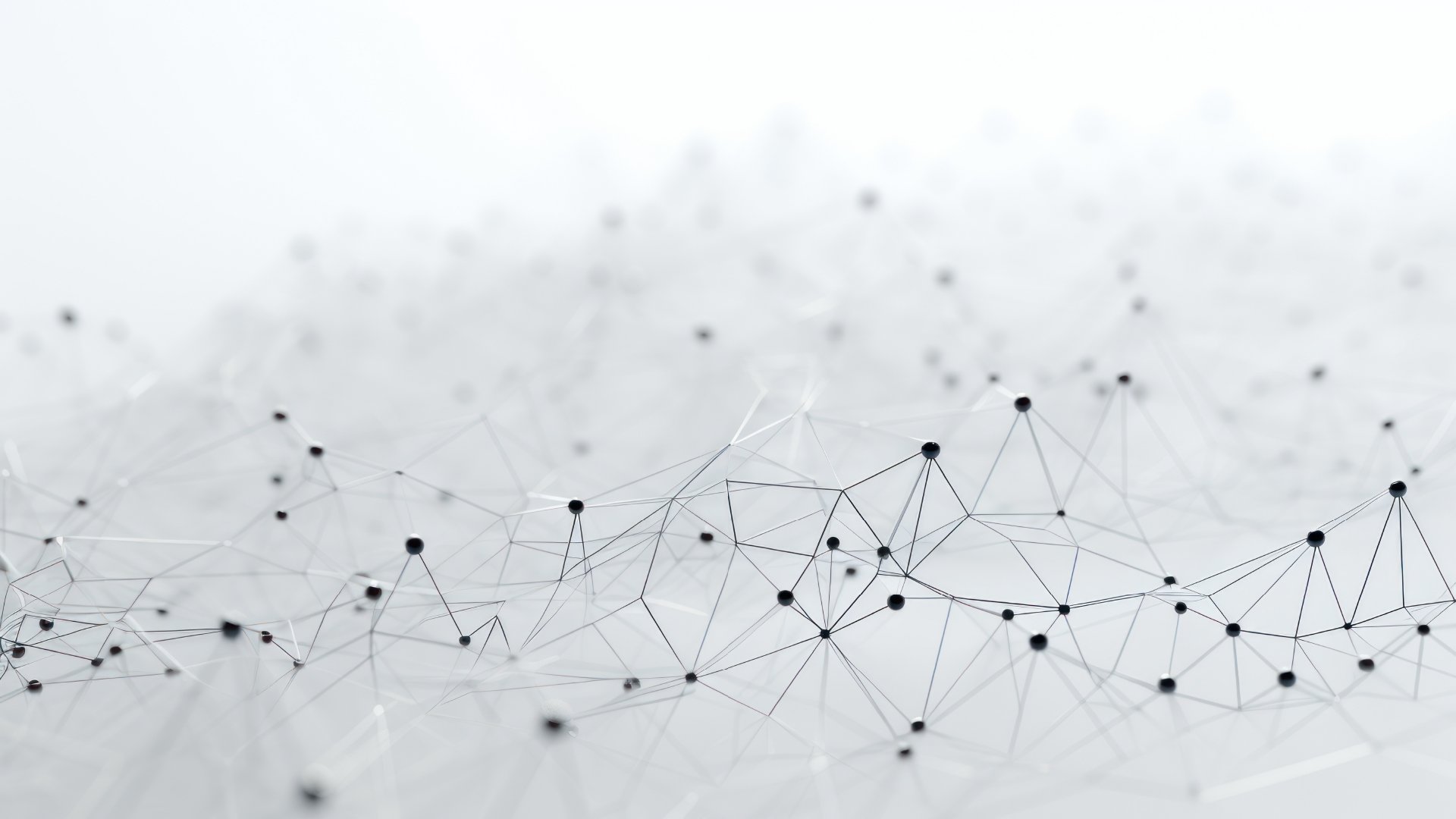
TRAC represents a new, modern approach to managing structural analytics.. but what is structural analytics?
Structural Analytics in Banking
Defining Structural Analytics
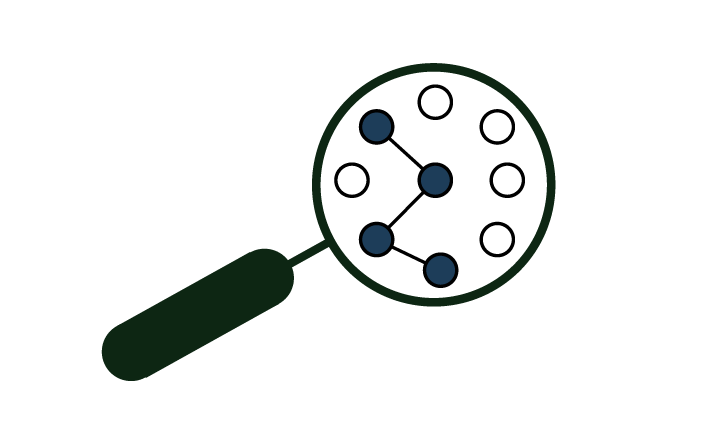
EXPLORATORY ANALYTICS
Identifying patterns, trends, and relationships in data to inform decisions and build hypotheses. Open-ended and iterative, using techniques like data mining, clustering, and visualisation.
PRIORITIES: Data interactivity, speed of prototyping and visualisation.
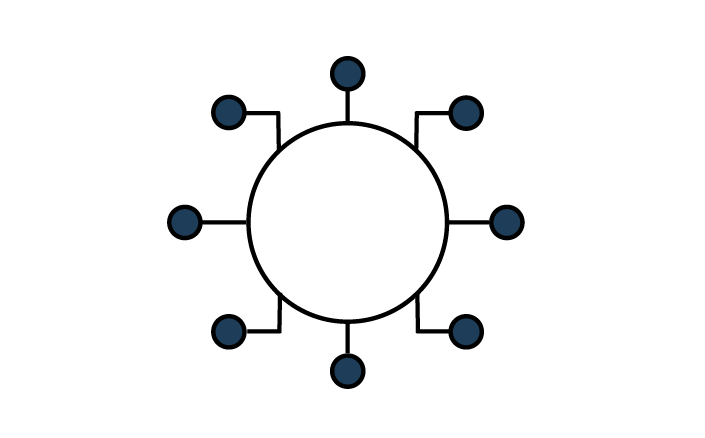
GENERATIVE ANALYTICS
Creating new data and content using AI/ML algorithms, where the system automatically adapts and adjusts as new data is introduced.
PRIORITIES: Scalability and the ability to automate pipelines and model training.
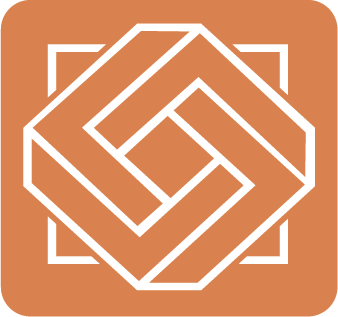
STRUCTURAL ANALYTICS
Applying complex, semi-rigid operations to generate data outputs that have predetermined business definitions and acceptance criteria.
PRIORITIES: Lineage, auditability and explainability.
Common Challenges With Structural Analytics
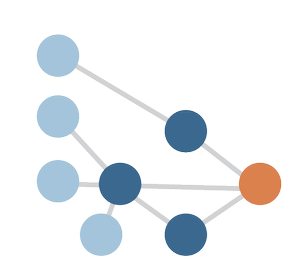
COMPLEX WORKFLOWS
Calculations built from several independently specified elements which require multiple sources, levels and types of data input.
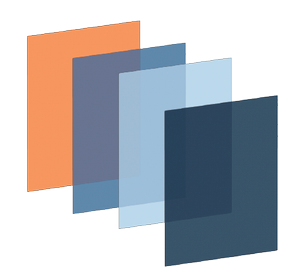
COMPLEX ANALYSIS
Need to explore the drivers and sensitivities of final outputs and model performance, possibly both in aggregate and on sub-populations .
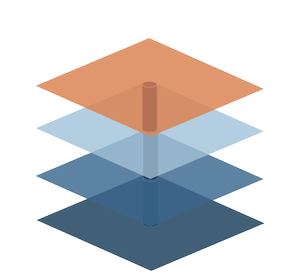
COMPLEX GOVERNANCE
Multiple control frameworks to satisfty, such as model governance, internal audit, financial control and data lineage reporting.
Take a Tour
See how TRAC's unique design solves all three of the challenges associated with structural analytics.